Thursday 29 April 2021
Live online meeting upon invitation, live streaming on Youtube
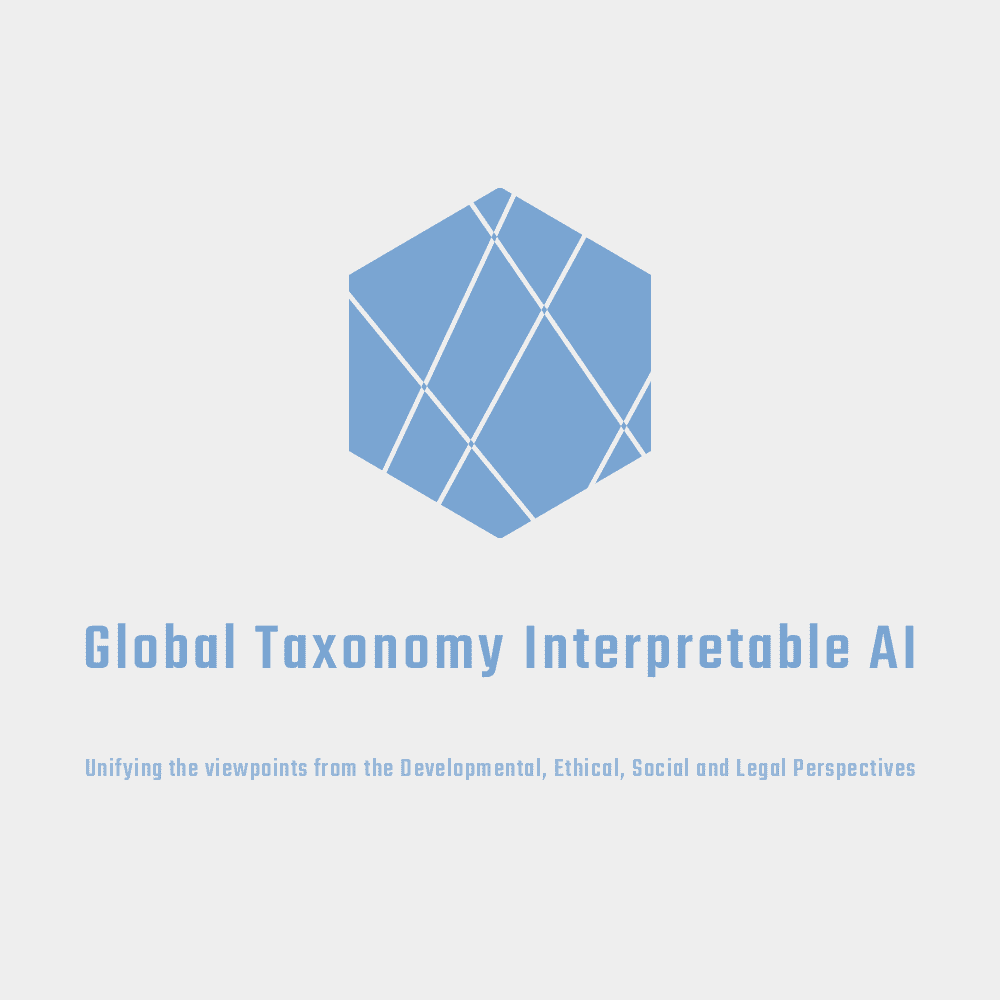
Interpretability analyses may have multiple scopes, e.g. debugging, justifying outcomes, proving the safety, reliability and fairness of the model, providing accountability. Such variety of objectives led to inconsistencies in the terminology of interpretable Artificial Intelligence. The words “interpretable”, “explainable”, “intelligible”, “understandable”, “transparent” and “comprehensible” have often been used interchangeably in the literature, causing confusion and different taxonomies.
A formal definition of interpretability was given by Doshi-Velez and Kim as that of “explaining or presenting in understandable terms to a human” the decisions of a machine learning system.
What does “understandable to a human” mean from the cognitive and psychological perspective? What are the legal constraints regarding the explanations? What are the ethical and social impacts of generating explanations and how can these meet the requirements of the technical development?
The goal of this workshop is to discuss all of these questions, among others. We aim at involving experts of different backgrounds to join forces towards obtaining a global viewpoint on interpretable AI that can then be presented in the joint publication “Common Viewpoint on Interpretable AI: Unifying the Taxonomy from the Developmental, Ethical, Social and Legal Perspectives”.
Program ( 29.04.2021 CEST time)
9:00-9:05
Lidia Dutkiewicz, Researcher at the Centre for IT and IP Law, KU Leuven
Welcome to the participants, presentation of the objectives
9:05-9:20
Mor Vered, Assistant Professor in the Laboratory of Dialogue Research, Monash University
9:20-9.35
Lidia Dutkiewicz, Researcher at the Centre for IT and IP Law, KU Leuven
9:35-9:50
Tobias Blanke, Professor in Social and Cultural Informatics at Kings College London and University of Amsterdam
9.50-10.00
Jean-Gabriel Piguet, Researcher at Hes-so Valais
10.05-10.20
Vijay Arya, Senior Researcher at IBM Research
10:20-10.35
Valeria Pulignano, Professor at the Center for Sociological Research at KU Leuven
10:35-10:50
Lode Lauwaert, Professor of Philosophy at KU Leuven
BREAK 15 minutes
11.05-12.00 Panel Session
Panel moderator
Mara Graziani, PhD student on Deep Learning Interpretability at HES-SO Valais and University of Geneva, Switzerland Taxonomy
Vijay Arya, Senior Researcher at IBM Research Michael Chromik, Managing Product Development, Strategic Partnerships and IT at CDTM and PhD candidate at LMU University Ethics and Legal
Wessel Reijers, Philosophy and Ethics at the European University Institute Katerina Yordanova, Researcher
Centre for IT & IP Law, KU LeuvenUse Case: Health
John O. Prior, Professor and Head of Nuclear Medicine and Molecular Imaging at Lausanne University Hospital, Switzerland BREAK 10 minutes
12:00 – 12.45 Organization of the paper manuscript and assignment of tasks.
Get updates on the event and paper
On the Speakers and Abstracts
Human Centred XAI and the Cognitive Sciences
Mor Vered
Mor Vered is a Lecturer at Monash University in the Faculty of IT. Her research interests lie in the interaction between humans and intelligent agents, where she works to incorporate lessons and inspirations from cognitive science, neuroscience and biology. She is a firm believer that only by focusing on interdisciplinary studies can we achieve results that can strongly impact human life. Her research focuses on Explainable AI, generating explanations built on cognitive theories, considering that these explanations need to be consumed by people and therefore taking into account human situation awareness models. Her research interests further include social human agent interaction, cognitive modeling and psychology.
To explain or to account? Accountability is all you need
Prof. Tobias Blanke
Tobias Blanke is Distinguished University Professor of Artificial Intelligence and Humanities at the University of Amsterdam. His academic background is in moral philosophy and computer science. Tobias’ principal research interests lie in the development and research of artificial intelligence and big data devices as well as infrastructures for research, particularly in the human sciences. Recently, he has also extensively published on ethical questions of AI like predictive policing or algorithmic otherings, as well as critical digital practices and the engagement with digital platforms. Tobias has authored three books, over 80 journal articles, conference papers and book chapters spanning traditional philosophy, computer science and digital studies. He has been an investigator on high-quality research grants well in excess of over €30m including the coordination of major European networks. Tobias’ monographs include most recently Digital Asset Ecosystems – Rethinking Crowds and Clouds, which offers a new perspective on the collaboration between humans and computers in global digital workflows. He is currently writing a book on the socio-economic position of AI called ‘Algorithmic Reason – the Governance of Self and Other’.
From right to safeguards. The explainability of automated decision-making under the General Data Protection Regulation (GDPR)
In the EU there are several legal frameworks which impose some forms of explainability when decisions are made with the help of an automated (AI) systems. Those include personal data protection law, consumer protection law, B2B rules (‘P2B Regulation’); or sector-specific rules. The main explainability obligations in the EU come from the General Data Protection Regulation 2016/679 (the ‘GDPR’). The core debate has primarily focused on whether or not the GDPR creates a right to explanation of an algorithmic decisions. In 2016 Goodman and Flaxman argued that the GDPR contained a ‘right to an explanation’ of algorithmic decision-making, whereas Wachter et al. in 2017 argued that a legally binding right to explanation does not exist. Others, like Selbst & Powles point out that whether one uses the phrase ‘right to explanation’ or not, the processors of personal data still have to give certain information to the recipients of decisions including “meaningful information about the logic involved, as well as (…) the envisaged consequences of such processing for the data subject” (art. 13(2f) and 14 (2g) of the GDPR). What this information constitutes in practice has been the subject of lively academic debate. De Streel et al. argue that there is no unique definition of explainability in law but different explainability levels. Some explainability requirements relate to the model while others relate to the (individual) decision. Moreover, WP29 Guidelines on Automated individual decision-making and Profiling provide that some explainability requirements relate to the features used by the model to adopt the decision (“the criteria relied on in reaching the decision”), while others go further and relate to the way the features are combined to make the decision (“the rationale behind the decision”). In addition, Kaminski & Malgieri argue that the focus on what information goes to whom when, is too narrow. Instead, they view algorithmic explanations not as static statements, but as a multi-layered process including systemic explanations, group explanations, and individual explanations. Despite many ambiguities one thing remains certain: there is an ever-growing need for an inter-disciplinary discussion between legal and other XAI communities on how the explainability requirements can be expressed in machine learning terms and what techniques make ML models complaint with the different levels of explainability required by law.
Lidia Dutkiewicz
Lidia Dutkiewicz is a legal researcher at KU Leuven Centre for IT & IP Law (CiTiP) focusing on data protection, media law, artificial intelligence (AI) and its ethical-legal implications for fundamental rights. Before joining KU Leuven, Lidia has been working in Brussels law firm specialized in entertainment and gambling law on aspects related to the GDPR, online advertising and technological challenges (e.g. loot boxes, AI). Lidia is a graduate of Brussels School of Competition programme on Law, Cognitive Technologies & Artificial Intelligence.
What is left of the « The mythos of model interpretability » (Lipton, 2016) ?
In this presentation, we will address Lipton’s famous article “The mythos of model interpretability”, discussing the main ethical objections it has raised since its publication in 2016.
Jean-Gabriel Piguet
One Explanation Does Not Fit All: A Toolkit and Taxonomy of AI Explainability Techniques
As machine learning algorithms make further inroads into society, calls are increasing from multiple stakeholders for these algorithms to explain their outputs. Moreover, these stakeholders, whether they be government regulators, affected citizens, domain experts, or developers, present different requirements for explanations. To address these needs, we introduce AI Explainability 360, an open-source software toolkit featuring eight diverse state-of-the-art explainability methods, two evaluation metrics, and an extensible software architecture that organizes these methods according to their use in the AI modeling pipeline. Additionally, we have implemented several enhancements to bring research innovations closer to consumers of explanations, ranging from simplified, more accessible versions of algorithms, to guidance material to help users navigate the space of explanations along with tutorials and an interactive web demo to introduce AI explainability to practitioners. Together, our toolkit can help improve transparency of machine learning models and provides a platform to integrate new explainability techniques as they are developed.
Vijay Arya
Vijay Arya is a Senior Researcher at IBM Research India and part of IBM Research AI group where he works on problems related to Trusted AI. Vijay has 15 years of combined experience in research and software development. His research work spans Machine learning, Energy & smart grids, network measurements & modeling, wireless networks, algorithms, and optimization. His work has received Outstanding Technical Achievement Awards, Research Division awards, and Invention Plateau Awards at IBM Research. His work on applying machine learning algorithms to improve the network model of distribution grids has been productized by IBM and implemented at power utilities in US. Before joining IBM, Vijay worked as a researcher at National ICT Australia (NICTA) and received his PhD in Computer Science from INRIA, France, and a Masters from Indian Institute of Technology (IIT) Delhi. He has served on the program committees of IEEE, ACM, and IFIP conferences, he is a senior member of IEEE & ACM, and has more than 60 conference & journal publications and patents.
Philosophical perspective on the opacity problem
In the context of AI, the lack of transparency is often seen as a moral problem; the requirement for technological systems is to be transparent. But what exactly is the problem with opacity? And is the overall condemnation of opacity correct? Is there no such thing as justified opacity?
Lode Lauwaert
Prof. Lauwaert works at the University of Leuven’s Institute of Philosophy, where he conducts research into violence and lectures philosophy of technology to engineers.
How Does AI Transparency Intersect with the Democratization of Work?
I will focus on Industry 4.0 technologies and indicate the main challenges it poses on workers quality of working life (QWLs), inclusive of autonomy (or worker discretionary interventions), deskilling (or upgrade skills) and setting (own) rules in organizational and production processes (transparency as information regarding decision making production processes). Within the realm of employment (and labour market) studies in sociology and economics there is an ongoing debate between those who see ‘taylorist’ strategies in which Industry 4.0 technologies deskill workers on the one hand, and ‘pragmatist’ ones in which firms develop employee skills to optimize Industry4.0 on the other hand. Hence, Industry 4.0 presents workers with risks but also opportunities.
Understanding whether the former or the latter (or a mix of both) prevails requires to systematically advance knowledge of factors and conditions influencing the future of work and workers and technology. I argue that advances in understanding participation (or involvement) structures and work organization as the way in which to build up democracy in a context of future work and technology requires to consider transparency of information and data exchange as strategies adopted by plant managers engineers and workplace representatives as the way to enhance firms’ positions in value chains as well as to augment employee skills and their shop-floor discretion. It is my contention that employees and their representative organizations can play a critical role in building up democratic process innovation as shop-floor employee interventions can help to overcome challenges related to the implementation of Industry 4.0 technologies.
Prof. Valeria Pulignano
Valeria Pulignano is an Italian sociologist, full Professor of Sociology at the University of Leuven (KU Leuven), Belgium, and author of numerous publications on comparative industrial relations, labour markets and employment in Europe. She was scientific director of the Center for Social Sociological Research (CeSO) at KU Leuven. She is Specialty Chief Editor of the “Work, Employment and Organization” section of Frontiers in Sociology,co-coordinator of the RN17 Work, Employment and Industrial Relations at the European Sociological Association (ESA) and principal investigator of the ERC Advanced Grant Research Project “Revolving Precariousness: Advancing the Theory and Measurement of Precariousness Across the Paid/Unpaid Continuum” (ResPecTMe).

The organizers
Hes-so Valais: Mara Graziani, Henning Müller, Vicent Andrearczyk, Davide Calvaresi, Jean-Gabriel Piguet
KUL: Lidia Dutkiewicz
IBM Research: Killian Levacher
Kings College London: Tobias Blanke
It is a pity that I will be busy on this date 😦
Is the meeting will be recorded and watched later on YouTube?
LikeLiked by 1 person
Hi Adil, thank you for your question. Yes, the recording of the workshop will be available on the MedGift Group YouTube channel to be watched later.
LikeLiked by 1 person